Remote sensing is entering a new era of time-series analysis. Short revisit times of satellites allow for monitoring of many areas across the globe on a weekly basis. However, there has been little exploration of deep learning techniques to leverage this new temporal dimension at scale. Especially, existing approaches have struggled to combine the power of different sensors to make use of all available information. In addition, large scale high quality change detection benchmarks are rare. To stimulate innovation in spatio-temporal machine learning, we have partnered up to propose a unique challenge centered around modeling multi-temporal land cover changes from Planetscope and Sentinel time series data.
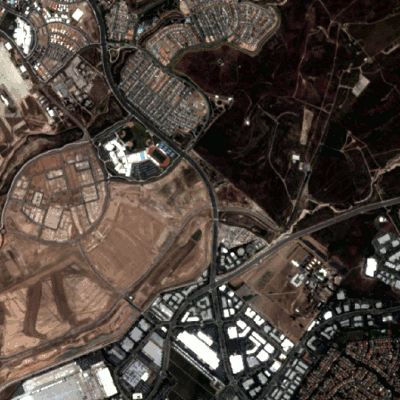
Dataset Details
- Images: Weekly, cloud-free, gap-filled Planetscope cubes from Jan 2018 to Dec 2019 from 75 AOIs [T=300+, H~=1024, W~=1024, C=RGB+NIR] with 3m resolution. These cubes share a new, unique processing level (Planet ‘Fusion’) as a Harmonized Landsat Sentinel (HLS) time series and are complemented by monthly Sentinel-2 cubes.
- Labels: Monthly high quality masks of seven land cover classes (LCC):
- Impervious surfaces
- Agricultural
- Forest
- Soil
- Water
- Wetlands
- Snow and ice
The challenge consists of two tracks in which participants are tasked to detect multi-temporal changes with few or no training labels. With this, we aim to incentivize the development of models without the necessity for large-scale annotations that is often not available in practice.
Unsupervised Binary Land Cover Change Detection
- Evaluation binary
- Multi-class information withheld to analyze heterogeneity in the performance depending on the type of change
- Track start : March 1, 2021
- Track end: April 15, 2021
Weakly-Supervised Multi-Class Change Detection
- Only a subset of training data has labels (multi-class)
- Evaluation: Multi-Class Change Detection
- Track start: April 15,2021
- Track end: June 1, 2021
How to participate
- Submit to the benchmark
- Send a 4-page abstract about your method
Prizes
- Win one of the three prestigious Beyond Fellowships (up to 8k€ per fellow) to visit the AI4EO Future Lab at the Technical University of Munich.
- Beyond Fellows visit TUM for 3-6 months to engage in cutting-edge research at the intercept of AI and EO with travel and accommodation costs covered. More information about the fellowships can be found here.
- The winning team of each track gets to select one fellow among their team and the third fellowship is awarded to the team with the most innovative approach.
- Challenge Start: March 1, 2021
- Challenge Deadline: June 1, 2021
- Winners awarded: at EarthVision 2021
- Xiaoxiang Zhu, German Aerospace Center (DLR) and Technical University of Munich (TUM)
- Laura Leal-Taixé, Technical University of Munich
- Giovanni Marchisio, Planet Labs
- Aysim Toker, Technical University of Munich
- Lukas Kondmann, DLR & TUM
- Andrés Camero Unzueta, DLR
- Jingliang Hu, TUM
- Chunping Qiu, TUM
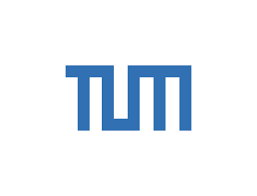
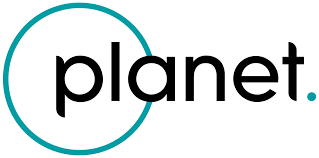
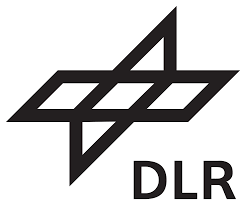